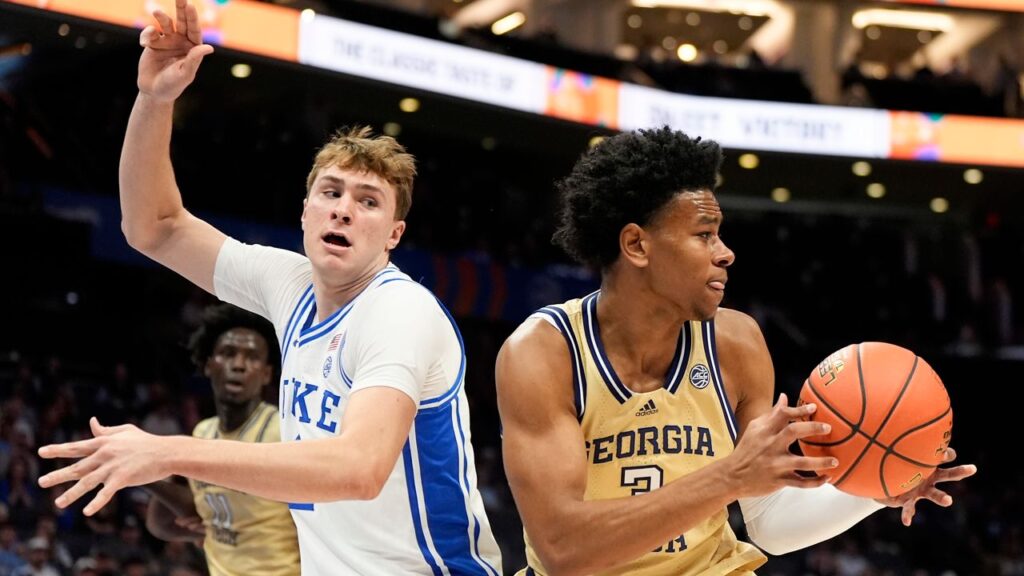
It might be challenging to forecast college basketball. These student data whizzes continued to attempt despite that.
The world of college basketball is notoriously unpredictable. The sport, rooted in tradition and ever-evolving, has seen countless upsets, Cinderella stories, and moments that defy even the most carefully laid out plans. For all the analyses, rankings, and projections, the NCAA Tournament often turns expectations on their head. Yet, amid this chaos, a group of data analysts—student whizzes, some might call them—have continued to push the boundaries of forecasting in college basketball, using advanced statistical models and algorithms to predict outcomes, identify trends, and forecast talent.
In a sport where surprises are the norm, these students are undeterred, constantly working to refine their models and enhance the accuracy of their predictions. They analyze player statistics, team dynamics, historical data, and even social media sentiment to create predictive models that they hope can replicate some of the unpredictability that defines college basketball. However, no amount of data can eliminate the unpredictability inherent in the game—especially when teams like a mid-major powerhouse or an underdog squad with raw potential can knock out a top-seed contender.
In this 2000-word article, we’ll delve into the ambitious efforts of these students, the challenges they face in forecasting college basketball, and what their data-driven insights tell us about the sport’s future.
The Rise of Data-Driven Analysis in College Basketball
Data analytics in sports is hardly a new concept. From baseball to football to basketball, teams have embraced technology to gain a competitive edge. In college basketball, the increasing prevalence of analytics has revolutionized how coaches scout talent, evaluate game strategy, and even recruit players. At the heart of this revolution are students—particularly those from fields like data science, mathematics, and engineering—who are applying their skills to solve one of the most complex puzzles in sports forecasting.
These student analysts are typically equipped with advanced computational tools that can sift through millions of data points, extracting insights from player performance metrics, advanced statistics like player efficiency ratings (PER) and box plus-minus (BPM), and historical trends. They combine these numbers with game footage analysis and team performance evaluations to build predictive models that aim to forecast outcomes as accurately as possible.
The tools at their disposal are powerful. Statistical models that predict everything from win probabilities to player efficiency and even potential draft positions can be fine-tuned based on continuous inputs and past performances. The rise of platforms like KenPom and team-specific data feeds has allowed students to leverage high-level analytics with unprecedented ease.
But even the most sophisticated models can’t entirely control for the many variables that make college basketball so unpredictable. This is where the challenge lies.
The Complexity of College Basketball
There’s no denying that college basketball presents a series of unique challenges when it comes to forecasting. The first is the sheer volatility of the sport itself. In the NCAA Tournament, a single-elimination format means that even the smallest of missteps or an off-day can be enough to derail a highly ranked team’s chances of advancing.
In addition, roster turnover in college basketball is high. Player injuries, transfers, and early departures for the NBA can all dramatically alter the dynamics of a team. Unlike professional leagues, where teams tend to have more stable rosters, college basketball teams are often in flux. The ever-changing landscape makes it difficult to account for the impact of new players, injuries, or changes in coaching philosophy.
The one-and-done rule also contributes to the challenge. Freshmen who enter the league after just one year of college ball can make an enormous impact, but their lack of experience and the rapid development of some players complicate things further. For every one-and-done star like Zion Williamson, who seemed like a surefire lottery pick from the moment he stepped onto the court, there are others who might disappoint due to inexperience or the pressure of NCAA competition.
Moreover, college basketball features a vast number of teams from different conferences, each with unique playing styles, coaching systems, and player talent. Predicting how a smaller, mid-major program will fare against a powerhouse team from a major conference is difficult, to say the least. Such unpredictability can throw off any forecast, regardless of the model’s sophistication.
Why Data Analysts Continue to Forge Ahead
Despite these challenges, data analysts—especially the student data whizzes—continue to push forward, seeking to unlock the mysteries of college basketball. Why? Because the potential rewards are massive. Accurate forecasts not only help teams make better strategic decisions but also provide insights for gamblers, media outlets, and fans who crave deeper engagement with the game.
Moreover, these students often view the challenge as an intellectual puzzle, an opportunity to apply their skills to a real-world scenario that demands creativity, persistence, and innovation. Many students see the application of data analysis to college basketball as a chance to make a meaningful impact on a sport they love, even if their forecasts don’t always hit the mark.
For some, the ultimate goal is a career in sports analytics, where success can lead to opportunities with NBA teams, data-focused firms, or even startups that cater to the sports industry. These ambitious students are not just forecasting for fun—they are building their portfolios and refining their models with the hope of one day joining the ranks of professional analysts.
Challenges of Forecasting the NCAA Tournament
One of the most important times for these student analysts is during the NCAA Tournament. March Madness represents the pinnacle of unpredictability in college basketball. The excitement surrounding the tournament is fueled by upsets, buzzer-beaters, and Cinderella runs. Teams like George Mason in 2006, Florida Gulf Coast in 2013, and Loyola-Chicago in 2018 have all defied expectations, making deep runs into the tournament, much to the dismay of the models that analysts spent weeks perfecting.
Part of the challenge in forecasting the tournament comes from the bracket format itself. In theory, a well-executed model might predict which teams have the best chances of making it to the Final Four or even winning the national championship. However, the single-elimination setup means that anything can happen in a one-game scenario. A star player could have an off-night, an underdog team could get hot, or an unexpected injury could derail a title contender’s journey.
Moreover, the models used by students often lack the “human” factors that come into play during the tournament. The intangible elements of college basketball—coaching adjustments, team chemistry, individual player motivations, and even crowd energy—are difficult to quantify but often make all the difference when it comes to a game’s outcome.
Additionally, there’s a psychological aspect to the NCAA Tournament that makes predictions even trickier. The pressure of the tournament setting can cause otherwise solid teams to crumble, while lesser-known teams may embrace the moment and rise to the occasion. Understanding the mental side of the game is critical, but even the most advanced algorithms can’t account for how players will handle the stress and excitement of the tournament.
How Students Tackle These Challenges
Given these complexities, students rely on a variety of approaches to improve the accuracy of their models. Some of the most common strategies include:
- Monte Carlo Simulations: These simulations model a wide variety of outcomes by running multiple scenarios based on historical data and various game variables. By simulating the tournament thousands or even millions of times, students can generate probability distributions that offer insights into potential outcomes.
- Machine Learning Algorithms: Machine learning, especially deep learning, has emerged as a powerful tool in sports forecasting. By training models on vast datasets, these algorithms can identify patterns and correlations that might be overlooked by traditional statistical models.
- Sentiment Analysis: Some students incorporate sentiment analysis from social media platforms like Twitter and Reddit, tracking the mood of fans, media outlets, and even players themselves. This can offer a glimpse into team dynamics and potential changes in motivation that might influence performance.
- Incorporating “Cinderella” Factors: Understanding what makes a lower-seeded team a potential “Cinderella” is essential. Some analysts focus on the non-statistical elements—momentum, coaching under pressure, or late-season performances—that can give an underdog an edge in the tournament.
- Hybrid Models: To capture both statistical data and human factors, some students create hybrid models that blend traditional analytics with subjective inputs from coaches, players, and analysts. These models might integrate expert opinions or account for momentum and other “gut feeling” elements that come into play.
The Future of College Basketball Forecasting
As technology continues to evolve and data analytics becomes even more sophisticated, the future of college basketball forecasting looks bright. Students, with their fresh perspectives and hunger for innovation, will remain at the forefront of this revolution. In the years to come, we may see models that can more accurately predict outcomes, uncover hidden talent, and even anticipate the next big upset before it happens.
Despite the unpredictability of the game, the students working tirelessly to understand it through data will continue to make strides in the realm of college basketball analysis. In the end, though, it is the inherent chaos and excitement of the sport—those surprise moments that no one can see coming—that will always be the true defining feature of March Madness and the college basketball experience. And perhaps that is why students continue to embrace the challenge of forecasting it: because, in the end, it’s all about the thrill of the unknown.